event
Thursday 21 Feb 2019: Deep learning driven mobile traffic analysis
Dr. Paul Patras - University of Edinburgh
IAIS Building/LT2 14:30-15:30
Abstract: Accurate understanding of mobile traffic at city scale, including the precise geographic distribution of the data traffic consumed by users and how this might evolve in the future is increasingly important for precision network engineering and demand-aware resource allocation. Gaining such knowledge is however difficult, because it requires large scale measurement collection using dedicated equipment and substantial storage capabilities. Furthermore, these measurements have to be subsequently aggregated at a central site, where insights are obtained though non-trivial post-processing. Commonly used interpolation tools make poor predictions about traffic consumption at precise locations, while traditional time-series based traffic forecasting methods largely estimate only trends and require continuous training.
In this talk I will present two techniques that harness the exceptional feature extraction abilities of deep learning to overcome these limitations and achieve precision mobile traffic analysis. First, I will introduce ZipNet-GAN, an original deep learning architecture that combines a Zipper Network and a Generative Adversarial Network, to infer narrowly-localised traffic consumption from coarse measurements, by uniquely exploiting spatio-temporal correlations inherent to mobile traffic. Results obtained by experimenting with a real-world data set will demonstrate that ZipNet-GAN infers traffic consumption with remarkable accuracy and up to 100x higher granularity as compared to standard probing, while outperforming existing data interpolation techniques. Second, I will reveal a Spatio-Temporal neural Network (STN) purposely designed for precise network-wide mobile traffic forecasting. By retraining this structure with prior predictions and combining its output with historical statistics, I will show that this enhanced approach can perform long-term traffic forecasting (up to 10 hours) using limited ground-truth observations (2 hours), achieving 61% smaller prediction errors as compared to widely used forecasting tools. Based on these early findings I will argue that deep learning has untapped potential to solve complex problems in the mobile networking domain.
Bio: Paul Patras is a Lecturer and Chancellor's Fellow in the School of Informatics at the University of Edinburgh, where he leads the Internet of Things Research Programme. He received his Ph.D. from University Carlos III of Madrid and held visiting research positions at the University of Brescia, Northeastern University, TU Darmstadt, and Rice University. His research interests include performance optimisation in wireless and mobile networks, applied machine learning, mobile traffic analytics, security and privacy, prototyping and test beds.
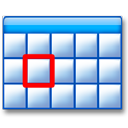