event
Thursday 15 Mar 2012: Hypothesis Testing and Bayesian Inference: New Applications of Kernel Methods
Dr. Arthur Gretton - Gatsby Computational Neuroscience Unit, University College London
Harrison 170 15:00-16:00
In the early days of kernel machines research, the "kernel trick" was
considered a useful way of constructing nonlinear learning algorithms
from linear ones, by applying the linear algorithms to feature space
mappings of the original data. More recently, it has become clear that
a potentially more far reaching use of kernels is as a linear way of
dealing with higher order statistics, by mapping probabilities to a
suitable reproducing kernel Hilbert space (i.e., the feature space is
an RKHS).
I will describe how probabilities can be mapped to kernel feature
spaces, and how to compute distances between these mappings. A
measure of strength of dependence between two random variables follows
naturally from this distance. Applications that make use of kernel
probability embeddings include:
* Nonparametric two-sample testing and independence testing in complex
(high dimensional) domains. In the latter case, we test whether text
in English is translated from the French, as opposed to being random
extracts on the same topic.
* Inference on graphical models, in cases where the variable
interactions are modeled nonparametrically (i.e., when parametric
models are impractical or unknown).
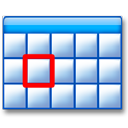